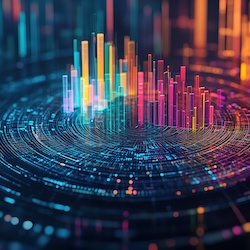
The research paper “Big Data and Predictive Analytics: A Systematic Review of Applications” offers an extensive overview of BDPA advancements from 2014 to 2023. Big data and predictive analytics (BDPA) have helped many businesses across industries process information to forecast trends and actionable insights effectively.
BDPA is, in fact, pivotal to an organization’s long-term sustainability. Teams must keep up with the evolving market scenarios quickly and make informed decisions to stay competitive. Additionally, extracting insights from real-world data is essential for streamlining operations, delivering better products or services, and enhancing customer experience.
In this article, we will examine the systematic review of BDPA applications to recognize their value, identify challenges, and identify areas for improvement.
Literature Review
“Big Data and Predictive Analytics: A Systematic Review of Applications” analyzes the advancements in BDPA over the past decade. The authors have identified seven key application domains—industrial, e-commerce, smart healthcare, smart agriculture, smart city, ICT, and weather.
While digital-first industries such as e-commerce are obvious, the remaining six domains reflect the broad scope of BDPA workflows and software solutions. Various procedures, such as data mining, statistical modeling, machine learning (ML), and other artificial intelligence (AI) techniques, are used to spot patterns in data and extract actionable insights.
Moreover, modern data pipelines allow data scientists to incorporate real-time analytics and deep learning to enhance the accuracy of predictions and forecasts. However, while there are innovative breakthroughs, BDPA is not without challenges.
Businesses and enterprises still struggle with managing large volumes of diverse data, especially when it is generated or gathered quickly. Additionally, existing roadblocks, such as ensuring data accuracy, protecting the analytics framework, and protecting user privacy, persist.
Avi Perez, co-founder and CTO of Pyramid Analytics, an industry-leading decision intelligence platform, dives into it further. In an interview with TechRound, Perez draws from his 16 years of BDPA experience to pinpoint three areas of friction:
- Answerability: Ensuring the AI models are capable of understanding and addressing the user’s queries
- Accuracy: The large language models (LLMs) must follow predefined guidelines and not hallucinate
- Usability: Intuitive chatbot interfaces can democratize data, enabling non-technical stakeholders to run analytics
The research paper acknowledges these issues and calls for continued research and innovation to overcome these limitations.
Methodology of Systematic Review
The authors of the paper followed a three-phase methodology while reviewing BDPA development in the past decade:
- Planning: Establishing a clear rationale and review protocols based on guidelines by Kitchenham and Brereton et al. This created precise research questions and evaluation metrics. A pilot study was conducted to ensure the efficacy of the guidelines and review protocols before examining papers.
- Execution: Developing comprehensive search strings with key terms such as “big data,” predictive,” and “forecasting,” along with platform names like Hadoop and Spark. These were used to find 1130 suitable articles in major databases like Google Scholar, ScienceDirect, Springer, and IEEE.
- Documentation: 109 articles were selected and classified into seven industry categories viz industrial, e-commerce, smart healthcare, smart agriculture, smart city, ICT, and weather. The authors extracted relevant insights and collated them in the paper.
The thorough approach in developing a comprehensive taxonomy of BDPA applications ensured an in-depth review, helping SMEs and industry experts enhance their understanding of relevant technologies.
Taxonomy of BDPA Applications
The systematic research paper has seven categories. Out of them all, the analytics applications and their scale of adoption in the e-commerce industry are remarkable.
For instance, the authors highlight the significance of leveraging big data analytics to predict stock market trends and credit risks. These financial tools are easily available in smartphone apps.
Similarly, product or service configuration recommendations, churn forecasting, and sentiment analysis are already widely adopted, reflecting the digital-first nature of online commerce and its agility. It is no surprise that e-commerce brands often edge out other companies from other industries in BDPA technology adoption.
The remaining six domains leverage BDPA technologies for a variety of purposes:
- Industrial
a. Enhancing production efficiency
b. Forecasting business outcomes
c. Real-time fault detection, maintenance tracking, and resource optimization
d. Improving supply chain performance and sustainability
- Smart healthcare
a. Early detection of conditions
b. Predicting patient readmissions
c. Simulating treatment responses
- Smart agriculture
a. Forecasting yields of harvests
b. Analyzing plant health to detect diseases
c. Precision agriculture to enhance irrigation, soil management, etc.
- Smart city
a. Traffic flow optimization
b. Elevating road safety
c. Infrastructure monitoring (safety, availability, and maintenance)
d. Crime prediction and surveillance
- Information and Communications Technologies (ICT)
a. Distributed architecture for large scale forecasting
b. New algorithms for modern datasets
c. Reasoning-enabled AI models for insights
- Weather
a. Forecasting weather parameters such as temperature and precipitation
b. Early warning systems for natural disasters like floods and typhoons
Current Challenges and Limitations
The implementation of BDPA faces both technical and ethical challenges.
From a technical perspective, large volumes of data from diverse sources can suffer from inconsistencies, inaccuracies, and incompleteness. Users interact with brands across platforms and media from different devices, complicating data collection further.
Consequently, teams may have to wrestle with data quality issues, such as missing values, noise, and errors. This makes analytics complex as professionals have to invest in data-cleaning techniques, which affects data democratization and drives up costs.
Ethical concerns also persist, particularly regarding user privacy and organizational data security. Using cloud-based BDPA solutions translates to organizations sending private data to proprietary LLMs, posing a security vulnerability.
Companies, particularly those in industries such as healthcare and finance, have to build tailored solutions to protect their clients’ interests. These tailored solutions include on-premise solutions and dedicated IT hardware such as servers and computers.
While these solutions are effective in enhancing security and privacy, they do pose a significant challenge — scaling up in a cost-effective manner. Businesses must maintain an in-house technical team that maintains and continually upgrades the internal digital infrastructure.
Future Directions and Innovations
Enhanced data quality and real-time collection of accurate data are essential areas of improvement for strengthening BDPA foundations for brands. It is critical to build efficient pipelines that record user data in real-time and transmit it safely to cloud or local servers.
Moreover, a majority of BDPA solutions help analyze past data and extract reports and insights from available datasets. While that is useful, fast-moving teams might need more.
Industry leaders, such as Pyramid Analytics, are already progressing toward diagnostic analytics. This goes a step beyond descriptive analytics, where LLMs recommend solutions to real-world problems.
Here, safety can be a concern as many enterprises in regulated industries, like pharmaceuticals, might be against sharing sensitive information with proprietary conversational AI models. Fortunately, there is a feasible workaround.
LLMs capture the user’s needs in natural language, which is then converted into machine-level SQL queries. These queries are then run on the databases in a secure environment, disconnected from the LLMs themselves.
After the queries retrieve the relevant information from the database, it is sent to the LLM. Finally, the AI chatbot converts the retrieved data into visualizations, insights, or recommendations, depending on the user’s needs.
This approach enables companies to get the best of both worlds. Teams can leverage LLMs’ advanced reasoning capabilities while upholding their stakeholders’ privacy. Another benefit of this tactic is that it works well with on-premise systems as well. Instead of connecting the internal database with the cloud, IT professionals can create an API that just sends the retrieved data to the servers.
Apart from speeding up analytics, it also scales up AI adoption in descriptive, predictive, and diagnostic use cases.
Conclusion
BDPA is indispensable in modern business environments where making data-driven decisions is pivotal.
The systemic review examines over a hundred papers highlighting various developments in BDPA technologies and strategies and segmenting them into multiple sectors, such as industrial and ICT.
While business intelligence and predictive analytics have made significant progress, further advancements are necessary to secure data, enhance forecast accuracy, and remain compliant.
Disclaimer: The author is completely responsible for the content of this article. The opinions expressed are their own and do not represent IEEE’s position nor that of the Computer Society nor its Leadership.