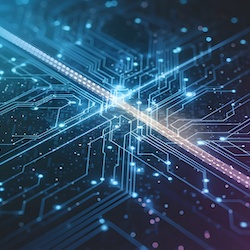
Generative AI refers to those algorithms that have the ability to create new content-image, text, music, or whatsoever-based on the patterns the algorithm learns from existing data. This technology has started to gain remarkable attention in recent times for its applications across different industries. On the other hand, edge computing is a form of distributed computing wherein data is processed close to its origin rather than relying on a centralized cloud server for processing exclusively. The convergence of generative AI and edge computing is revolutionizing how organizations are taking advantage of data and making real-time decisions.
The Need for Real-Time Processing
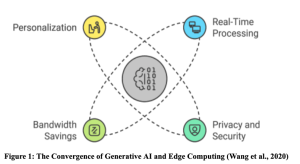
Real-time data processing is among the major reasons generative AI will be deployed at the edge, as illustrated in figure 1. Most applications require instant reactions and decisions, per input data. In the event of autonomous vehicles, for instance, generative AI would create synthetic scenarios in real time that would help the car know its way through difficult driving conditions. The processing of information locally on edge devices reduces latency significantly, thus allowing quicker decision-making-a factor very important in safety and efficiency.
Improving Privacy and Security
Health or financial information is regularly subject to privacy concerns within the industry. To this effect, it will be beneficial for the organization to consider employing edge computing in the running of generative AI applications by processing data locally rather than over a network where it can be intercepted or otherwise exposed. Keeping the data close to its source diminishes much of the associated risk with data in transit. This can also have very positive effects on security and helps to gain trust among a growing number of users sensitive about how their information is used.
Save Bandwidth
Most generative AI models are power-consuming, which naturally requires high bandwidth. As models based on cloud connectivity increase in usage, they put pressure on the networks, thus raising costs. By offloading a portion of the processing to edge devices, it frees up network congestion and reduces latency related to transferring large volumes of data between devices and cloud servers. This shift in processing facilitates efficient utilization of bandwidth while ensuring responsiveness in mission-critical applications.
Personalization Through Edge-Based Generative AI
Another critical benefit for generative AI at the edge is the delivery of experiences that are truly personalized in real time. For example, in retail environments, AI would analyze consumer behavior in real time and present product suggestions to customers based on their preferences; this increases user engagement, suggesting what users need at the exact moment they need it.
Implementation Challenges
While generative AI can be deployed today at the edge, significant challenges are felt mainly because of resource constraints that come with the edge devices. Unlike powerful cloud servers hosting big models, a lot of edge devices have limited processing ability and memory, as shown in figure 2. Specifically, model pruning-the removal of non-essential components, quantization reduction of numerical precision, and knowledge distillation-transfer of knowledge from bigger models, among other techniques, are being employed to make models efficient for deployment in resource-constrained environments.
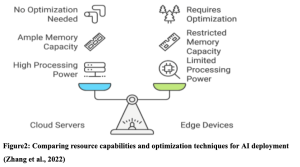
Conclusion
Combining generative AI with edge computing is the necessary leap forward in how businesses get work done across varied industry segments. Thus, as organizations continue to demand faster decision-making-keeping bandwidth constraints and privacy concerns in mind-the acceptance rates of generative AI deployments are most likely to increase at the edge. This evolution is ripe not only to create more operational efficiencies but also to imagine new possibilities for innovation across varied industries.
References
Wang, X., Han, Y., Leung, V. C., Niyato, D., Yan, X., & Chen, X. (2020). Edge AI: Convergence of edge computing and artificial intelligence (pp. 3-149). Singapore: Springer.
Zhang, W., Zeadally, S., Li, W., Zhang, H., Hou, J., & Leung, V. C. (2022). Edge AI as a service: Configurable model deployment and delay-energy optimization with result quality constraints. IEEE Transactions on Cloud Computing, 11(2), 1954-1969.
Disclaimer: The author is completely responsible for the content of this article. The opinions expressed are their own and do not represent IEEE’s position nor that of the Computer Society nor its Leadership.