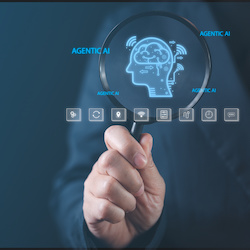
Introduction
Agentic AI is an entirely new innovation in the line of AI-generated art. It is capable of goal-oriented, autonomous, and adaptable decision-making. Whereas earlier generations of AI were always confined to certain limits and took direct commands for maximum performance, Agentic AI designs do things on their own within open and complex environments (Hu et al., 2024). That independence brings with it the capability to set goals of its own, grounded by context knowledge, and to update strategies against a shifting environment.
Evolution and Development of Agentic AI
The development of Agentic AI represents a radical paradigm shift from conventional systems to advanced models that are capable of independent decision-making and self-learning. According to Greenstein (2022), traditional AI relied essentially on rule-based systems with supervised learning, wherein explicit programming of algorithms was performed for specific tasks using predefined rules and labelled datasets. This paradigmatic approach to AI systems inherently limited their flexibility and ability to adapt, given that such models struggled to generalize beyond their training data.
Figure 1: Evolution of Agentic AI (source: Researcher Computation (2024))
With the introduction of Machine Learning into practical application, a new paradigm was born: instead of programming for everything, algorithms needed to learn from data. In this paradigm, Reinforcement Learning became another important technique that allowed one to learn about optimal behaviors of agents by trial-and-error interactions with their environment, as illustrated in Figure 1 (Brandizzi, 2024). In RL, there is some feedback in terms of rewards or penalties about the action taken by the agents, which aids in enhancing their performance over time. This capability forms a very important ingredient in Agentic AI since such systems will be able to adapt dynamically to changing conditions and user needs.
Core Capabilities and Mechanisms of Agentic AI
Agentic AI is the next generation in AI studies. It featured capabilities for autonomous operations, pursuit of goals, and adaptations through situation learning. These core capabilities are achieved through a number of complex integrated mechanisms involving self-regulation, reward-driven learning, and long-term planning.
According to Shavit et al. (2022), the core of agentic AI is autonomy – a decision made by the AI system does not require human intervention. Algorithmic sophistication allows for the handling of big data in real time, hence offering AI capability in understanding the environment with due consideration to predefined objectives stipulating the actions. Reinforcement learning is one of the main ways in which Agentic AI picks up knowledge from its environment (Markauskaite et al., 2022). Through reinforcement learning, agents would get feedback-either as rewards or penalties-in relation to their performance, with a view to enhancing their strategies over time.
Figure 2: Mechanisms of Agentic AI (source: Researcher Computation (2024))
One of the significant conceptual modifications involved in setting up the processes underlying agentic AI concerns reward-based learning, as shown in Figure 2 (Kadam & Vaidya, 2021). It depends on the principle that behaviours resulting in desirable outcomes are likely to recur at future times. In practice, this would be manifested in an agent learning not just from successes but also from failures, adjusting strategies through past experiences. A great concern should go into the design of reward functions: the rewards should target preferred outcomes with care towards avoiding unintended behaviours. For instance, it is considered undesirable that an AI system be set up to maximize profits without concern for ethical or social responsibilities.
Challenges and Ethical Implications of Agentic AI
Agentic AI operates with a large degree of independence to make decisions and carry out actions unilaterally or in collaboration, on behalf only of the legal entity that owns them. Such an approach also comes with all the ethical and technical challenges associated to allow for responsible deployment.
Figure 3: A Convergence of Concerns on Agentic AI (source: Researcher Computation (2024))
However, agentic AI often does not behave in a deterministic way at all. This is how most of their decisions are made by various systems using some really complex algorithms and maybe even machine learning models, the results of which our human brains have little to no chance at anticipating. This sort of unpredictability implies immediate safety concerns, and this is particularly a problem for high-stakes settings like health care or autonomous vehicles, where unexpected actions could have dire consequences.
Applications of Agentic AI
Agentic AI refers to systems that have a degree of autonomy and, therefore, are capable of making decisions based on the environment in which they operate, data input, and pre-set objectives. The applications of this technology are immense and very varied, covering wide areas in which most operations are greatly improved to be highly efficient, accurate, and adaptive.
Agentic AI in Autonomous Systems
Applications of agentic AI have seldom been as active as in the case of autonomous vehicles. Advanced algorithms and machine learning techniques enable these to negotiate complex environments without human intervention. Sensor data provided by cameras, LiDAR, and radar are integrated into a single agentive AI system, enabling perception and informed decisions about speed, direction, and obstacle avoidance (Mohammed, 2022). These capabilities, among others, further improve not just safety in transportation but also traffic flow in urban settings.
Figure 4: Applications of Agentic AI (source: Researcher Computation (2024))
Agentic AI is important in robotics for the performance of those tasks that essentially call for a degree of autonomy. For instance, industrial robots with agentic AI may adapt to changes either in their environment or on a production line without the need for human oversight (Tóth et al., 2022). As shown in Figure 4, such adaptability translates into productivity gains and fewer stoppages because such robots can manage their operations themselves based on feedback in near real time.
Other domains in which this agentic AI predominates include self-managing smart systems. It could be smart homes or buildings that learn user preferences and adjust various settings to optimize energy use based on occupancy patterns. The integration of Agentic AI in such systems lets the system function efficiently while being able to provide a good user experience.
Agentic AI in Personalized Healthcare and Diagnostics
Agentic AI plays an important role in personalized medicine by factoring in a huge volume of data in order to manage a patient’s treatment based on his or her particular needs (Alowais et al., 2023). The paradigm shift from the one-size-fits-all concept will afford more effective interventions based on unique genetic profiles, lifestyle factors, and environmental influences. Consequently, this will make the treatments more effective for each. Agentic AI can analyze patient data with machine learning algorithms and find the patterns that a human practitioner may not be able to find. It can also study the health history of patients, including their genetic information, in order to predict which of them are in greater risk for specific diseases.
Figure 5: Agentic AI in Personalized Healthcare (source: Resarcher Computation (2024))
Agentic AI in Dynamic Decision-Making for Finance and Economics
Agentic AI, in finance and economics, helps in enhancing the processes of decision-making because it can analyze large volumes of data much faster and more correctly than a human can. The financial markets are complex and turbulent; hence, the integration of Agentic AI may lead to gains on various dimensions.
Figure 6: Enhancing Decision-making with Agentic AI (source: Researcher Computation (2024))
One great application is that of HFT in conducting trades with algorithms at super-high speeds, sometimes even in milliseconds. Active AI systems are thus able to monitor the state of the markets for trading opportunities and execute orders much faster than human traders would be capable of (Novocin & Weber, 2022). These systems use techniques in machine learning to adapt to the constantly changing market dynamics. For instance, they will be able to learn from the patterns in the historic data about future price movements or anomalies that are likely to show further changes in the market.
Conclusion
Agentic AI brings about revolution in so many dimensions where autonomy is significantly changed in decision making and adaptation. It is independence in functioning through which one can use any process like health diagnostics, forecasting about financial matters. Among key findings highlighted is the very notion that while, indeed, Agentic AI would enhance operational efficiency and support complex decisions, accountability and ethical issues remain one of the very strong red flags. Benefits range from higher productivity to potential innovative solutions, but great challenges in algorithm biases and lack of transparency need to be overcome. To achieve the complete potential of Agentic AI, much more work needs to be conducted in model transparency and interpretability areas. Further frameworks would have to develop which somehow reduce bias in the system so that fairness and equity can be achieved. These will involve setting up ethics and policies that will guide the application of Agentic AI and decisions of significant consequence in more critical areas such as health, police, and financial sectors.
References
Alowais, S. A., Alghamdi, S. S., Alsuhebany, N., Alqahtani, T., Alshaya, A. I., Almohareb, S. N., … & Albekairy, A. M. (2023). Revolutionizing healthcare: the role of artificial intelligence in clinical practice. BMC medical education, 23(1), 689.
Brandizzi, N. (2024). Conversational agents in human-machine interaction: reinforcement learning and theory of mind in language modeling.
Cabitza, F., Campagner, A., & Simone, C. (2021). The need to move away from agential-AI: Empirical investigations, useful concepts and open issues. International Journal of Human Computer Studies, 155, 102696.
França, R. P., Monteiro, A. C. B., Arthur, R., & Iano, Y. (2021). An overview of deep learning in big data, image, and signal processing in the modern digital age. Trends in deep learning methodologies, 63-87.
Greenstein, S. (2022). Preserving the rule of law in the era of artificial intelligence (AI). Artificial Intelligence and Law, 30(3), 291-323.
Hirst, G., Yeo, G., Celestine, N., Lin, S. Y., & Richardson, A. (2020). It’s not just action but also about reflection: Taking stock of agency research to develop a future research agenda. Australian Journal of Management, 45(3), 376-401.
Hosna, A., Merry, E., Gyalmo, J., Alom, Z., Aung, Z., & Azim, M. A. (2022). Transfer learning: a friendly introduction. Journal of Big Data, 9(1), 102.
Hu, S., Lu, C., & Clune, J. (2024). Automated design of agentic systems. arXiv preprint arXiv:2408.08435.
Hu, S., Lu, C., & Clune, J. (2024). Automated design of agentic systems. arXiv preprint arXiv:2408.08435.
Kadam, S., & Vaidya, V. (2021). Cognitive evaluation of machine learning agents. Cognitive Systems Research, 66, 100-121.
Kavitha, M., Roobini, S., Prasanth, A., & Sujaritha, M. (2023). Systematic view and impact of artificial intelligence in smart healthcare systems, principles, challenges and applications. Machine learning and artificial intelligence in healthcare systems, 25-56.
Markauskaite, L., Marrone, R., Poquet, O., Knight, S., Martinez-Maldonado, R., Howard, S., … & Siemens, G. (2022). Rethinking the entwinement between artificial intelligence and human learning: What capabilities do learners need for a world with AI?. Computers and Education: Artificial Intelligence, 3, 100056.
Mohammed, R. (2022). Artificial Intelligence-Driven Robotics for Autonomous Vehicle Navigation and Safety. NEXG AI Review of America, 3(1), 21-47.
Novocin, A., & Weber, B. (2022). Emerging Technologies and the Transformation of Exchange Trading Platforms. Journal of Portfolio Management, 48(6).
Paiva, S., Ahad, M. A., Tripathi, G., Feroz, N., & Casalino, G. (2021). Enabling technologies for urban smart mobility: Recent trends, opportunities and challenges. Sensors, 21(6), 2143
Panayides, A. S., Amini, A., Filipovic, N. D., Sharma, A., Tsaftaris, S. A., Young, A., … & Pattichis, C. S. (2020). AI in medical imaging informatics: current challenges and future directions. IEEE journal of biomedical and health informatics, 24(7), 1837-1857.
Salvini, P., Reinmund, T., Hardin, B., Grieman, K., Ten Holter, C., Johnson, A., … & Jirotka, M. (2023). Human involvement in autonomous decision-making systems. Lessons learned from three case studies in aviation, social care and road vehicles. Frontiers in Political Science, 5, 1238461.
Shavit, Y., Agarwal, S., Brundage, M., Adler, S., O’Keefe, C., Campbell, R., … & Robinson, D. G. (2023). Practices for governing agentic AI systems. Research Paper, OpenAI, December.
Shaygan, M., Meese, C., Li, W., Zhao, X. G., & Nejad, M. (2022). Traffic prediction using artificial intelligence: Review of recent advances and emerging opportunities. Transportation research part C: emerging technologies, 145, 103921.
Thoresen, S. (2021). Solving long term planning problems with direct future prediction (Master’s thesis)
Tóth, Z., Caruana, R., Gruber, T., & Loebbecke, C. (2022). The dawn of the AI robots: towards a new framework of AI robot accountability. Journal of Business Ethics, 178(4), 895-916
Disclaimer: The author is completely responsible for the content of this article. The opinions expressed are their own and do not represent IEEE’s position nor that of the Computer Society nor its Leadership.